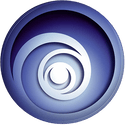
Ubisoft Exploring Generative AI, Could Revolutionize NPC Narratives
Have you ever dreamed of having a real conversation with an NPC in a video game? Not just one gated within a dialogue tree of pre-determined answers, but an actual conversation, conducted through spontaneous action and reaction? Lately, a small R&D team at Ubisoft's Paris studio, in collaboration with Nvidia's Audio2Face application and Inworld's Large Language Model (LLM), have been experimenting with generative AI in an attempt to turn this dream into a reality. Their project, NEO NPC, uses GenAI to prod at the limits of how a player can interact with an NPC without breaking the authenticity of the situation they are in, or the character of the NPC itself.
Considering that word—authenticity—the project has had to be a hugely collaborative effort across artistic and scientific disciplines. Generative AI is a hot topic of conversation in the videogame industry, and Senior Vice President of Production Technology Guillemette Picard is keen to stress that the goal behind all genAI projects at Ubisoft is to bring value to the player; and that means continuing to focus on human creativity behind the scenes. "The way we worked on this project, is always with our players and our developers in mind," says Picard. "With the player in mind, we know that developers and their creativity must still drive our projects. Generative AI is only of value if it has value for them."
Considering that word—authenticity—the project has had to be a hugely collaborative effort across artistic and scientific disciplines. Generative AI is a hot topic of conversation in the videogame industry, and Senior Vice President of Production Technology Guillemette Picard is keen to stress that the goal behind all genAI projects at Ubisoft is to bring value to the player; and that means continuing to focus on human creativity behind the scenes. "The way we worked on this project, is always with our players and our developers in mind," says Picard. "With the player in mind, we know that developers and their creativity must still drive our projects. Generative AI is only of value if it has value for them."