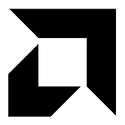
AMD also Announces Radeon Instinct MI8 and MI6 Machine Learning Accelerators
AMD also announced the Radeon Instinct MI8 and MI6 Machine Learning GPUs based on Fiji and Polaris cores, respectively. These parts comprise the more "budget" part of the still most certainly non-consumer oriented high-end machine learning lineup. Still, with all parts using fairly modern cores, they aim to make an impact in their respective segments.
Starting with the Radeon Instinct MI8, we have a Fiji based core with the familiar 4 GBs of HBM1 memory and 512 GB/s total memory bandwidth. It has 8.2 TFLOPS of either Single Precision of Half Precision floating point performance (so performance there does not double when going half precision like its bigger Vega based brother, the MI25). It features 64 Compute Units.
The Radeon Instinct MI6 is a Polaris based card and slightly slower in performance than the MI8, despite having four times the amount of memory at 16 GBs of GDDR5. The likely reason for this is a slower bandwidth speed, at only 224 GB/s. It also has less compute units at 36 total, with a total of 2304 stream processors. This all equates out to a still respectable 5.7 TFLOPs of overall half or single precision floating point performance (which again, does not double at half precision rate like Vega).
Starting with the Radeon Instinct MI8, we have a Fiji based core with the familiar 4 GBs of HBM1 memory and 512 GB/s total memory bandwidth. It has 8.2 TFLOPS of either Single Precision of Half Precision floating point performance (so performance there does not double when going half precision like its bigger Vega based brother, the MI25). It features 64 Compute Units.
The Radeon Instinct MI6 is a Polaris based card and slightly slower in performance than the MI8, despite having four times the amount of memory at 16 GBs of GDDR5. The likely reason for this is a slower bandwidth speed, at only 224 GB/s. It also has less compute units at 36 total, with a total of 2304 stream processors. This all equates out to a still respectable 5.7 TFLOPs of overall half or single precision floating point performance (which again, does not double at half precision rate like Vega).