Tuesday, March 12th 2024
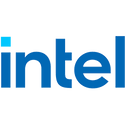
Intel Gaudi2 Accelerator Beats NVIDIA H100 at Stable Diffusion 3 by 55%
Stability AI, the developers behind the popular Stable Diffusion generative AI model, have run some first-party performance benchmarks for Stable Diffusion 3 using popular data-center AI GPUs, including the NVIDIA H100 "Hopper" 80 GB, A100 "Ampere" 80 GB, and Intel's Gaudi2 96 GB accelerator. Unlike the H100, which is a super-scalar CUDA+Tensor core GPU; the Gaudi2 is purpose-built to accelerate generative AI and LLMs. Stability AI published its performance findings in a blog post, which reveals that the Intel Gaudi2 96 GB is posting a roughly 56% higher performance than the H100 80 GB.
With 2 nodes, 16 accelerators, and a constant batch size of 16 per accelerator (256 in all), the Intel Gaudi2 array is able to generate 927 images per second, compared to 595 images for the H100 array, and 381 images per second for the A100 array, keeping accelerator and node counts constant. Scaling things up a notch to 32 nodes, and 256 accelerators or a batch size of 16 per accelerator (total batch size of 4,096), the Gaudi2 array is posting 12,654 images per second; or 49.4 images per-second per-device; compared to 3,992 images per second or 15.6 images per-second per-device for the older-gen A100 "Ampere" array.There is a big caveat to this, and that is the results were obtained using the base PyTorch; Stability AI admits that with the TensorRT optimization, A100 chips produce images up to 40% faster than Gaudi2. "On inference tests with the Stable Diffusion 3 8B parameter model the Gaudi2 chips offer inference speed similar to Nvidia A100 chips using base PyTorch. However, with TensorRT optimization, the A100 chips produce images 40% faster than Gaudi2. We anticipate that with further optimization, Gaudi2 will soon outperform A100s on this model. In earlier tests on our SDXL model with base PyTorch, Gaudi2 generates a 1024x1024 image in 30 steps in 3.2 seconds, versus 3.6 seconds for PyTorch on A100s and 2.7 seconds for a generation with TensorRT on an A100." Stability AI credits the faster interconnect and larger 96 GB memory as making the Intel chips competitive.
Stability AI plans to implement the Gaudi2 into Stability Cloud.
Sources:
Stability AI, Wccftech
With 2 nodes, 16 accelerators, and a constant batch size of 16 per accelerator (256 in all), the Intel Gaudi2 array is able to generate 927 images per second, compared to 595 images for the H100 array, and 381 images per second for the A100 array, keeping accelerator and node counts constant. Scaling things up a notch to 32 nodes, and 256 accelerators or a batch size of 16 per accelerator (total batch size of 4,096), the Gaudi2 array is posting 12,654 images per second; or 49.4 images per-second per-device; compared to 3,992 images per second or 15.6 images per-second per-device for the older-gen A100 "Ampere" array.There is a big caveat to this, and that is the results were obtained using the base PyTorch; Stability AI admits that with the TensorRT optimization, A100 chips produce images up to 40% faster than Gaudi2. "On inference tests with the Stable Diffusion 3 8B parameter model the Gaudi2 chips offer inference speed similar to Nvidia A100 chips using base PyTorch. However, with TensorRT optimization, the A100 chips produce images 40% faster than Gaudi2. We anticipate that with further optimization, Gaudi2 will soon outperform A100s on this model. In earlier tests on our SDXL model with base PyTorch, Gaudi2 generates a 1024x1024 image in 30 steps in 3.2 seconds, versus 3.6 seconds for PyTorch on A100s and 2.7 seconds for a generation with TensorRT on an A100." Stability AI credits the faster interconnect and larger 96 GB memory as making the Intel chips competitive.
Stability AI plans to implement the Gaudi2 into Stability Cloud.
8 Comments on Intel Gaudi2 Accelerator Beats NVIDIA H100 at Stable Diffusion 3 by 55%
I don't really know all that much about the data center specific hardware like this though, it could just be than Intel has the better overall solution and you know what, good on them too.
There are several versions of NVIDIA x100-series accelerators, that is Non-PCI-e and PCI-e, and it is Not clear what was actually used.
NVIDIA A100 accelerators are almost 4-year-old ( released in 2020 ).
Tests using PyTorch instead of TensorRT can Not be considered seriously for NVIDIA x100-series accelerators.
The 1st test for Intel Gaudi2 uses twice more accelerators than NVIDIA accelerators ( 32 vs. 16 / final results, that is images per sec, should be normalized ).
>>...Stability AI admits that with the TensorRT optimization, A100 chips produce images up to 40% faster than Gaudi2...
Once again, Tests using PyTorch instead of TensorRT can Not be considered seriously for NVIDIA x100-series accelerators.
Also first I've heard of stable diffusion 3. Hope that one is good, because a lot of people are still on 1.5, having skipped 2 and not had the processing power to tune XL