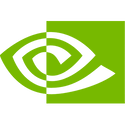
NVIDIA Blackwell Platform Boosts Water Efficiency by Over 300x - "Chill Factor" for AI Infrastructure
Traditionally, data centers have relied on air cooling—where mechanical chillers circulate chilled air to absorb heat from servers, helping them maintain optimal conditions. But as AI models increase in size, and the use of AI reasoning models rises, maintaining those optimal conditions is not only getting harder and more expensive—but more energy-intensive. While data centers once operated at 20 kW per rack, today's hyperscale facilities can support over 135 kW per rack, making it an order of magnitude harder to dissipate the heat generated by high-density racks. To keep AI servers running at peak performance, a new approach is needed for efficiency and scalability.
One key solution is liquid cooling—by reducing dependence on chillers and enabling more efficient heat rejection, liquid cooling is driving the next generation of high-performance, energy-efficient AI infrastructure. The NVIDIA GB200 NVL72 and the NVIDIA GB300 NVL72 are rack-scale, liquid-cooled systems designed to handle the demanding tasks of trillion-parameter large language model inference. Their architecture is also specifically optimized for test-time scaling accuracy and performance, making it an ideal choice for running AI reasoning models while efficiently managing energy costs and heat.
One key solution is liquid cooling—by reducing dependence on chillers and enabling more efficient heat rejection, liquid cooling is driving the next generation of high-performance, energy-efficient AI infrastructure. The NVIDIA GB200 NVL72 and the NVIDIA GB300 NVL72 are rack-scale, liquid-cooled systems designed to handle the demanding tasks of trillion-parameter large language model inference. Their architecture is also specifically optimized for test-time scaling accuracy and performance, making it an ideal choice for running AI reasoning models while efficiently managing energy costs and heat.